Analytics and AI are profoundly transforming how businesses and governments engage with consumers and citizens. Across many industries, high value transformative use cases in personalized medicine, predictive maintenance, fraud detection, cybersecurity, logistics, customer engagement and more are rapidly emerging. In fact, AI adoption alone has grown an astounding 270% in the last four years and 40% of organizations expect it to be the leading game changer in business[1]. However, for analytics and AI to become an integral part of an organization, numerous deployment challenges with data and infrastructure must be overcome – data volumes (50%), data quality and management (47%) and skills (44%)[2].
In addition, many companies are beginning to use hybrid cloud and multi-cloud computing models to knit together services to reach higher levels of productivity and scale. Today, large organizations leverage almost five clouds on average. About 84% of organizations have a strategy to use multiple clouds[3].
IBM Cloud Pak for Data is an end-to-end Data and AI platform that reduces complexity, increases scalability, accelerates time to value and maximizes ROI with seamless procedures to extend to multiple clouds. While Cloud Pak for Data and can run on any public or private cloud, it is also modular and composable allowing enterprises to embrace just the capabilities that they need on-premises. So, it is truly a hybrid multi-cloud platform.
Recently, IBM announced enhancements to IBM Cloud Pak for Data (Version 3.5). These enhancements can be broadly grouped into 2 key themes: Cost Reduction and Innovation to drive digital transformation. Customers can drive down costs through automation, consolidated management and an integrated platform. On the innovation front, Accelerated AI, Federated Learning, improved governance & security and an expanded ecosystem are the key focus areas. In this blog, we primarily focus on the value of Federated Learning.
Federated learning (also known as collaborative learning) is a machine learning technique that trains an algorithm across multiple decentralized edge devices or servers with local datasets, without transferring them ( Figure 1). The data stays local and it allows for executing deep learning algorithms while preserving privacy and security. This approach is different from traditional centralized machine learning techniques where all the local datasets are uploaded to one server and deep learning ML algorithms are executed on this aggregated dataset.

Figure 1: Comparison of Federated Learning and a Standard Approach
Federated learning enables multiple actors to build a common, robust machine learning model without sharing data, thus maintaining data privacy, data security, data access rights and access to heterogeneous data. Many industries including defense, telecommunications, IoT, healthcare, manufacturing, retail and others use federated learning and getting significant additional value from their AI/ML initiatives.
For IBM Cloud Pak for Data, this additional value can be quantified using the Cabot Partners Total Value of Framework.
High Level TVO Framework for Federated learning
TVO analysis is an ideal avenue to quantify and compare the value of Federated Learning compared to the standard approach for Machine Learning. In the TVO analysis, the Total Value (Total Benefits – Total Costs) of IBM Cloud Pak for Data solution with Federated Learning is compared against IBM cloud Pak for Data solution without Federated Learning
The TVO framework (Figure 2) categorizes the interrelated cost/value drivers (circles) for Analytics by each quadrant: Costs, Productivity, Revenue/Profits and Risks. Along the horizontal axis, the drivers are arranged based on whether they are primarily Technical or Business drivers. Along the vertical axis, drivers are arranged based on ease of measurability: Direct or Derived.
The cost/value drivers for Analytics are depicted as circles whose size is proportional to the potential impact on a client’s Total Value (Benefits – Cost) of Ownership or TVO as follows:
- Total Costs of Ownership (TCO): Typical costs include: one-time acquisition costs for the hardware and deployment, and annual costs for software, maintenance and operations. For the case without Federated Learning, the costs associated with data transfer to a central repository need to be considered.
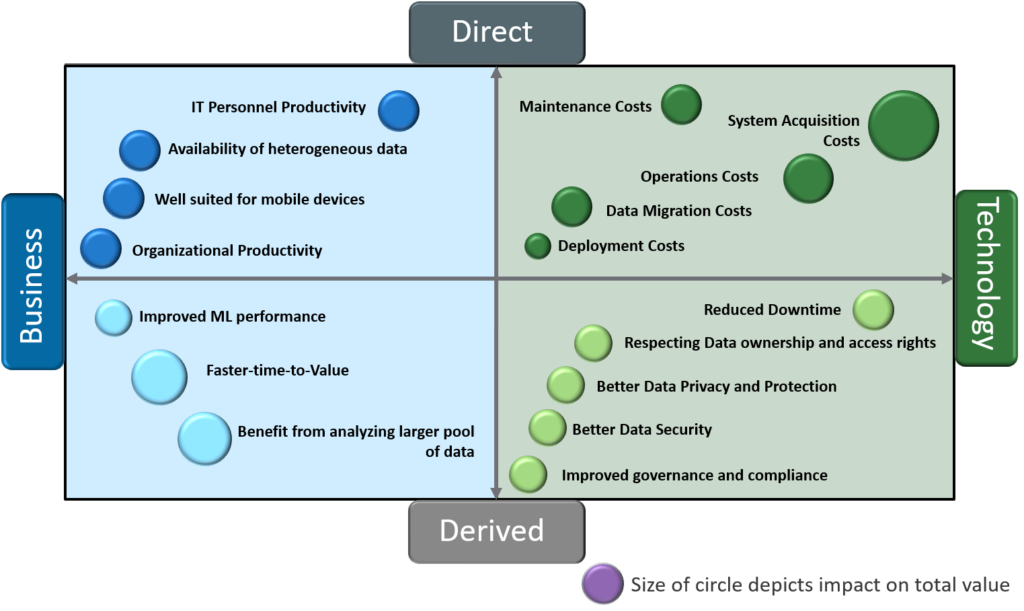
Figure 2: TVO Framework for Federated Learning with Cost/Value Drivers
- Improved Productivity: The TVO model quantifies the value of productivity gains of data scientists, data engineers, applications developers and the organization. It should also consider the value associated with the availability of additional heterogeneous data due to Federated Learning. Federated Learning enables mobile phones to collaboratively learn a shared prediction model while keeping all the training data on device, decoupling the ability to do machine learning from the need to store the data in the cloud and the value associated with this innovation need to be considered for applicable cases.
- Revenue/Profits: Benefit of Federated Learning is access to a large pool of data, resulting in increased machine learning performance, while respecting data ownership and privacy. Faster time to value with better performance results in greater innovation and better decision-making capabilities which spur growth, revenues and improve profits.
- Risk Mitigation: Federated Learning enables multiple actors to build a common, robust machine learning model without sharing data, thus allowing users to address critical issues such as data privacy, data security, data access rights which also allows for improved governance and compliance.
The above Framework is a simplified pictorial look of TVO analysis. In a rigorous TVO analysis, which is a major offering of Cabot Partners, the elements of the framework are quantified and expressed in easily understandable business terms. In addition, the analysis can be expanded include other innovation features.
Conclusions
IBM, recently announced enhancements to IBM Cloud Pak for Data (version 3.5). The enhancements focus primarily on cost reduction and Innovation to drive digital transformation. A major element of innovation is Federated Learning. As detailed above, Federated Learning amplifies the value of IBM Cloud Pak for Data through:
- Lower costs – no costs associated with data migration to a central database location
- Availability of heterogeneous data improves the quality of ML models
- Access to larger pool of data resulting in increased ML performance
- Improved security
- Multiple actors to build a common robust ML model without sharing data, thus allowing to address critical issues such as data privacy and data access rights
[1] https://futureiot.tech/gartner-ai-adoption-growing-despite-skills-shortage/
[2] Ritu Jyoti, “Accelerate and Operationalize AI Deployments Using AI – Optimized Infrastructure”, IDC Technology Spotlight, June 2018
[3] RightScale® STATE OF THE CLOUD REPORT 2019 from Flexera™